Model Memory
Model Memory is a validated set of reference data and documents that Affinda's models use to enhance accuracy over time. By leveraging Retrieval-Augmented Generation (RAG), Model Memory enables Affinda to dynamically reference previously validated documents, allowing the model to predict future documents more accurately without requiring constant retraining.
Key Benefits of Model Memory
- Continuous improvement – The model improves over time by referencing validated documents, ensuring better predictions without the need for extensive manual adjustments.
- No need for constant prompt engineering – Instead of adjusting prompts for every case, Model Memory provides a more scalable approach to improving AI performance.
- High accuracy for repeated formats – The system quickly learns and perfects the extraction for recurring document formats, such as invoices from a particular supplier.
Options for Selecting Documents for Model Memory
Users can control how documents are added to Model Memory through the Workspace Settings in the Affinda app. There are three options:
- Auto (Default Option) – Affinda intelligently selects which documents are added.
- Manual – No documents from the workspace are automatically added to Model Memory, even after validation.
- Always – Every validated document from the workspace is added to Model Memory.
Auto is the recommended option for most Workspaces intended for ongoing model learning. Under these settings, document formats not already well-covered in Model Memory are intelligently selected, ensuring:
- The memory does not grow excessively large, making it easier to audit.
- The model remains efficient by avoiding redundant examples of well-represented formats.
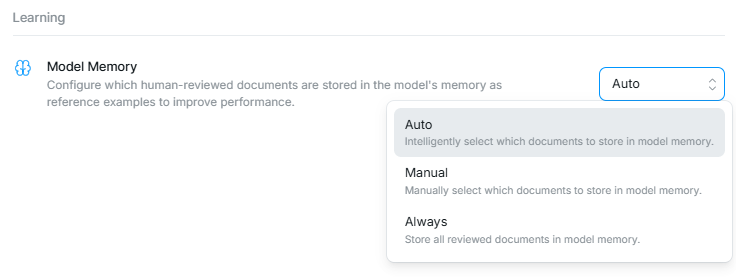
How Model Memory is Used
Affinda utilizes a Fingerprinting algorithm to identify documents similar to a newly uploaded document. This approach ensures that the most relevant validated examples from Model Memory are used to enhance extraction accuracy.
How It Works:
- When a new document is uploaded, Affinda’s Fingerprinting algorithm scans Model Memory to find sufficiently similar documents.
- If relevant documents are found, one or more of these validated examples are provided to the model.
- The model uses these documents to improve extraction accuracy and consistency.
By using this method, Model Memory provides the model with real-world examples, reducing the likelihood of errors and continuously improving the accuracy of document processing.
Importance of Highly Accurate Data in Model Memory
Since Model Memory directly references individual documents and their validated data, the accuracy of annotated documents is critical. Incorrect or low-quality annotations can lead to:
- Confusion in model predictions – The model may reference incorrect information, leading to erroneous outputs.
- Propagation of mistakes – Since Model Memory applies learnings from past documents, any errors will be replicated in future extractions.
Best Practices for Ensuring High-Quality Data
- Use the Auto mode for document selection – This ensures a manageable and auditable dataset.
- Correct errors promptly – Any errors discovered downstream should be corrected in the Affinda app to maintain data integrity.
- Establish clear validation guidelines – If multiple team members validate documents, create clear annotation standards to prevent inconsistencies, especially in cases where ambiguity exists.
By following these best practices, organizations can maximize the effectiveness of Model Memory and achieve unparalleled accuracy in document processing.
Updated about 1 hour ago